When i did passed out I did perceive that engineer should have
knowledge of his particular faculties all the fundamentals so
he/she/other can solve the problems and make it new level of upgradation.
but still to make it simple and abstract three fields has been
classified as Software,Device/Hardware and Networking.
and all this happen because skills and career option could be
simplified as software need more logic and calculation and understanding
regarding skill. in hardware/device he/she/other needs to be more
accurate with circuits, assembling of ICs and soldering otherwise there
will be huge cost in repairing or de-assembling or might be dis-soldering
will happen. and in network all needs to know about operations and
operations regarding protocols and protocols regarding configuration and
configuration regarding tools.
On this level everything looks fine because all of this three fields
required of relevant but still not highly dependent knowledge on each
other. as software is mostly about programming, hardware/device is
mostly about circuits making and networking is all about troubleshooting
and configurations.
but what hacks me that how classifications in each of that fields
happen that sounds completely ridiculous are most of computer engineers
are become marketer of specific company or organization or become lazy
professionals that does not have any more passion to learn or spin off
to new or other relevant technologies. or Narrow minded people that just
want to stick with known things and mindset. as In software field now
there are no more software engineers or software developers there are
.net develops or Java developer or PHP developers.
If you have worked on java and you go for .net than in most of firms
you will be told that we don't work on Java and we want expert on .net.
call you if there any requirement for java.
I know nower days most of
firms in India are just a code shop. not driven by true solution
oriented or product/service oriented company service ,problem and
product lies outside of India and only code is written on their firm
more you write code more you get paid. and if any specific code is
already stacked by leaving of developer than new developer is needed to
continue write that code. and ASAP start to continue. so money flow not
get stopped. and that is why more than 86% code is getting written in India but still stay a lot behind in actual engineering.
I think in software industries there is need to understand actual
engineering aspect instead of just selling code. and if there will be an approach to give solution to particular audience to provide
service/product than there will be the need of specific alter techniques
and need of understanding to be explored as Medium to small level software did not need to get distributed on multi-layer architecture
so that can be easily deployed on Apache using PHP or ruby and
development+learning both will be faster than creation and deployment
on JAVA/C#.
And there are lots of API are there to define easier way to complete
the project smooth and rapidly. and for content management there is no
need to build any project from the scratch. just need to configure the
CMS as per requirement.
And this same thing is happening in networking side as well windows server administrator and Redheat server administrator.
And nower days things are getting more worse spring developer and Asp.net devXpress developer or ASP.net mvc developer.
hope that computer engineering still be art of solving
through mathematical,logical,Automation,Communication,connectivity and storage
and management problems. instead of just making configuration or writing code for some money.
Thanks for Read till the end. share your comments.
The key thing i understand about authority is that. Authority
is only exist becouse in organization each and every person have
responsibility of one or multiple task and the one who has most
experience and who is highly paid for that has more responsibility. and
to fullfill his responsibility he need total cooperation of people. and
to achive that he has been assigned some level of authorities.
As far as I understand any Tech/Engineering based organization
following department mostly exist in almost all of the organization and
each have their responsibility.
1. Adminstrative/Management/Executive:-
this is exist because each and every organization have their own
goal/aim/vision as well as purpose. and people who are members of this
department are responsible for defining what must to do and where should
now organization flow must go on with spirit.
2. Sales/Marketing with Account:-
this exist because every organization need to define their
market/social value to achive their goal/aim/vision and so first what
should be product/services`s cost that must be defined and one which
class of socity/market this product is going to make impact and how much
big ipact this product could do at what level of depth. once this
research has been done Sales/Marketing stratagy along with bussiness
model should be defined.
3. Tech and Support / Operations.:-
this exist because none of the vision/goal/aim or purpose could be
achieved without particular technologies (If tech/engineering based
organization) and this is highly special skilled job. so to define new
solution strong team of technical people is most necessary thing. so
this department is necessary for How or which way goal/purpose/aim or
vision is going to get achieved.
so in moderan corporate organization i belief that responsibility has
been distributed properly but what making me harsh is how authority has
been distributed. all the authorities except recruiting staff based on
specialization has been centralized and have in hand of Head of
Management/Administrative/Executive department. each and every
organization `s any decision that is good for organization`s progress
must be passed through management. all the rules and regulation that is
good for only one department and bad for other still followed by all
department.
and still if any department work is not get done properly than
responsible person is Head of that Particular department. I know there
will be time of conflict where two department head will be against each other point. but for that management/executive/administrative
department just need to be mediator to solve their conflict by defining
priority and effectiveness. there is no need to keep all the authority
to centralize.
and of course i am not stating here that Head of
Administrative/Executive/Management should not have authority to fire or
analyze all the department. he/she must have rights for firing any head
person of that particular department. but why all the authorities have
been centralized for MD/CEO/Executive director or Chairman.
isn't this bureaucrat-ism is hazardous for organization in sense of achieving its goal/vision/aim or purpose.
Hello Readers,
i
am writing this because i am feeling now so much passion to writing
this story that did make huge impact on my mind this is the story that
is also responsible for open my eye about what is engineering exactly
and which kind of aspect every engineers should have. write me any
feedback that comes to your mind. after reading this.
This
is the story about one man who wants to startup his own timber business
and looking for some person that do cut some lumber in the forest. and
he had two candidate one is Labor with Muscular Physics and another is
recently passed out diploma Cutting and Fabrication Engineer with not
that much Physical Strength. After taking an interview of both of them.
He become confuse that which side he should go for.
because
Labor was asking just for 5000 rupees per month and that Engineer was
asking for 10,000 rupees per month he did not able to take decision
rationally so he decided to hire both of them just for experiment and
find conclusion that which side he should go for. and he assign task to
both of them to cut the lumber with axe.
1st day labor did cut 7 logs and Engineer did 3 only
2nd day labor did cut 7 logs again and Engineer did 3 only
3rd day labor did cut 6 logs again and Engineer did 4 only
4th day as same as 3rd day
.....
after 14 days passed
15th day labor did cut 3 and Engineer did 7
After getting this shocking reverse observation that businessman did call both of them and ask what happening with them.
Then labor did replied he is doing 3 times more hard work then before but
still he can't able to score because his time is not running well, or
god is not with his or may be his luck is not with him.
Then
he did ask same question to Engineer then he reply that in starting
days he become tired early so he was not able to score higher. but then
he got practiced so he able to improve his score.
But still confusion of that business man did not cleared so he did switch their axes with each other.
and
then both score was near to equal Engineers score was one down then
Labor and he said that axe blade was not sharp enough to cut the lumber
so he did take long time to make it sharpen by rubbing it between stone.
and than that Business man ask same question to the Labor and he
replied that he did not instructed to do so.
Then
that business man was cleared that if he want more profit with aspect
of long time then he should hire Engineers then Labor.
After then engineer did suggest to use Chainsaw with electric motor instead of Axe so he can do better.
So
this should be an Aspect of Engineering to upgrade the present
situation with more felicities with applying scientific knowledge in progressive direction ( ofcours with limitation that it should not harm an environment i am aware of forest devastation due to timber business and
Minamata pollution and etc...).
I
am writing this because nower days in India Engineering is just to go
college and study subject and passing examinations and score in that for
earning degrees instead of Gaining knowledge and apply it for do or
create something that matters.
This Story did help me to earning Engineering Aspect I hope it help you to all as well.
Thanks For Reading it Till end.
please share your reviews
Engineering, enterprise, and social impact are interconnected fields that collectively contribute to societal advancement and quality of life. This article explores the relationship between these three domains, illustrating how engineering innovations drive enterprise success and generate significant social impact.
Engineering: The Catalyst for Innovation
- Technological Advancements
- Innovation and Development: Engineering is the backbone of technological innovation, leading to the creation of new products, processes, and solutions that address various challenges.
- Example: The development of renewable energy technologies such as solar panels and wind turbines, which help reduce carbon emissions.
- Problem-Solving Approach
- Engineering Mindset: Engineers apply a systematic approach to problem-solving, which is essential for developing efficient and effective solutions in various fields.
- Example: Civil engineers designing resilient infrastructure to withstand natural disasters, thereby enhancing public safety.
Enterprise: Harnessing Engineering for Economic Growth
- Commercialization of Innovations
- Bringing Ideas to Market: Enterprises play a crucial role in bringing engineering innovations to market, transforming prototypes into commercially viable products and services.
- Example: A startup leveraging biotechnology to develop and market advanced medical diagnostics tools.
- Economic Development
- Job Creation and Wealth Generation: Enterprises that harness engineering advancements contribute to economic development by creating jobs, generating wealth, and stimulating further innovation.
- Example: The rise of the tech industry, driven by engineering innovations, leading to the creation of millions of jobs and significant economic growth.
- Operational Efficiency
- Improving Processes: Enterprises utilize engineering principles to optimize operations, reduce costs, and improve efficiency, enhancing overall business performance.
- Example: Manufacturing companies implementing lean production techniques to minimize waste and increase productivity.
Social Impact: Enhancing Quality of Life
- Addressing Societal Challenges
- Solving Real-World Problems: Engineering-driven enterprises develop solutions that address critical societal challenges, such as access to clean water, healthcare, and education.
- Example: Engineers Without Borders working on projects to provide clean drinking water in developing countries.
- Sustainable Development
- Environmental Stewardship: Engineering innovations contribute to sustainable development by creating eco-friendly technologies and practices that reduce environmental impact.
- Example: The development of electric vehicles to reduce greenhouse gas emissions and dependence on fossil fuels.
- Improving Public Health
- Healthcare Advancements: Engineering plays a pivotal role in advancing healthcare through the development of medical devices, diagnostic tools, and treatment methods.
- Example: The invention of minimally invasive surgical instruments that reduce recovery times and improve patient outcomes.
Image: Surgeons using advanced minimally invasive surgical tools
The Synergistic Relationship
- Collaboration and Partnerships
- Interdisciplinary Collaboration: The collaboration between engineers, enterprises, and social organizations leads to the development of holistic solutions that maximize social impact.
- Example: A public-private partnership between tech companies and non-profits to provide internet access in underserved regions.
- Innovation Ecosystem
- Creating an Innovation Ecosystem: The synergy between engineering, enterprise, and social impact fosters an innovation ecosystem where ideas are rapidly developed, commercialized, and scaled to benefit society.
- Example: Innovation hubs and incubators that support startups with engineering backgrounds to develop solutions with significant social impact.
Conclusion
The relationship between engineering, enterprise, and social impact is fundamental to creating a better future. Engineering drives innovation, enterprises harness and commercialize these innovations, and the resulting products and services generate substantial social impact. By fostering collaboration across these domains, we can address pressing global challenges, promote sustainable development, and enhance the quality of life for people worldwide.
Bitcoin, often hailed as the revolutionary digital currency, promised to transform the financial landscape with its decentralized nature and blockchain technology. However, despite its initial promise, Bitcoin has largely failed to become a stable and widely accepted form of currency. Instead, it has morphed into a speculative asset, often likened to gambling. This article explores the reasons behind Bitcoin's failure to fulfill its original vision and its transformation into a speculative instrument.
Volatility and Instability
- Extreme Price Fluctuations
- Volatile Market: Bitcoin's value is highly volatile, with dramatic price swings driven by market sentiment, speculation, and regulatory news. This instability makes it impractical for everyday transactions.
- Example: In 2017, Bitcoin's price skyrocketed from around $1,000 to nearly $20,000, only to crash to around $3,000 in 2018, illustrating its unpredictable nature.

- Lack of Price Stability
- Unpredictable Value: For a currency to be effective, it needs to maintain a stable value. Bitcoin's unpredictable fluctuations deter its use as a reliable medium of exchange or store of value.
- Example: Merchants are reluctant to accept Bitcoin due to the risk of significant value loss between the time of sale and the time they can convert it to fiat currency.
- Lack of Price Stability
- Unpredictable Value:
For a currency to be effective, it needs to maintain a stable value.
Bitcoin's unpredictable fluctuations deter its use as a reliable medium
of exchange or store of value.
- Example:
Merchants are reluctant to accept Bitcoin due to the risk of significant
value loss between the time of sale and the time they can convert it to
fiat currency.
- Legal Issues and Fraud
- Association with Illegal Activities: Bitcoin's anonymity has made it a preferred medium for illegal activities, including money laundering and black market transactions. This association has led to negative perceptions and stricter regulations.
- Example: The notorious Silk Road marketplace, which facilitated illegal drug sales using Bitcoin, highlighted the currency's use in illicit activities.
- Merchant Acceptance
- Low Merchant Adoption: Despite initial enthusiasm, relatively few merchants accept Bitcoin for everyday transactions. This limited acceptance reduces Bitcoin's utility as a currency.
- Example: Major companies like Tesla and Microsoft initially accepted Bitcoin but later reversed their decisions, citing volatility and environmental concerns.
- Transaction Speed and Costs
- Scalability Issues: Bitcoin transactions can be slow and expensive, especially during periods of high demand. This inefficiency makes it less attractive for everyday use compared to traditional payment methods.
- Example: During peak times, Bitcoin transaction fees can skyrocket, and confirmation times can extend to hours, making it impractical for quick purchases.
- Speculative Nature
- Investment Over Use: Bitcoin is primarily viewed as a speculative investment rather than a functional currency. Investors buy Bitcoin hoping its value will increase, rather than to use it for transactions.
- Example: The media frequently covers Bitcoin as an investment opportunity, focusing on potential profits rather than its utility as a currency.
- Market Manipulation
- Influence of Whales: A small number of large holders, or "whales," can significantly impact Bitcoin's price, leading to market manipulation and reinforcing its speculative nature.
- Example: Sudden large transactions by whales can cause significant price movements, further deterring its use as a stable currency.
Bitcoin's journey from a revolutionary digital currency to a speculative asset highlights the challenges it faces in becoming a stable and widely accepted medium of exchange. Its extreme volatility, regulatory hurdles, limited adoption, and speculative nature have hindered its potential to be the new age of currency. Instead, Bitcoin has become more akin to a gambling solution, attracting investors seeking high-risk, high-reward opportunities. For Bitcoin to fulfill its original promise, it must overcome these challenges and achieve greater stability, regulatory clarity, and widespread acceptance.
Engineering thinking is a systematic approach to problem-solving that draws on principles of engineering to address complex issues efficiently and effectively. It involves critical and analytical thinking, creativity, and a structured methodology to design, test, and implement solutions.
Key Components of Engineering Thinking
Problem Identification and Analysis
- Defining the Problem: Clearly identifying the problem to understand its scope and impact.
- Analyzing Requirements: Determining the needs and constraints associated with the problem to guide the solution process.
Research and Data Collection- Gathering Information: Collecting relevant data and researching existing solutions and technologies.
- Benchmarking: Comparing current solutions to industry standards and best practices to identify areas for improvement.
Idea Generation and Conceptualization- Brainstorming: Encouraging creative thinking to generate a wide range of potential solutions.
- Concept Development: Refining ideas into feasible concepts that can be further analyzed and tested.
Design and Prototyping- Detailed Design: Creating detailed plans and specifications for the chosen solution.
- Prototyping: Building prototypes or models to test and refine the design.
Testing and Evaluation- Testing: Conducting rigorous tests to evaluate the performance and reliability of the solution.
- Analysis and Feedback: Analyzing test results and gathering feedback to make necessary adjustments and improvements.
Implementation and Deployment- Final Production: Transitioning from prototype to final product or solution, ensuring it meets all requirements.
- Deployment: Implementing the solution in the real-world context and monitoring its performance.
Iteration and Continuous Improvement- Feedback Loop: Continuously collecting feedback and performance data to identify areas for further enhancement.
- Iterative Design: Making iterative improvements to refine and optimize the solution over time.
Applications of Engineering Thinking in Everyday Life
Home Improvement Projects: Using systematic problem-solving to plan and execute home renovations efficiently.
Personal Finance Management: Applying analytical skills to create and maintain budgets, and to make informed investment decisions.
Workplace Efficiency: Implementing engineering principles to streamline workflows, enhance productivity, and foster innovation.
Benefits of Engineering Thinking
Enhanced Problem-Solving Skills: Developing a structured approach to tackle complex issues.
Improved Creativity and Innovation: Encouraging creative thinking and the exploration of diverse solutions.
Better Decision-Making: Making informed decisions based on thorough analysis and testing.
Increased Efficiency and Effectiveness: Streamlining processes and optimizing resources.
ConclusionEngineering thinking equips individuals with the skills and mindset to approach problems methodically and creatively. Whether in professional settings or everyday life, adopting engineering thinking can lead to more effective solutions and continuous improvement.
By understanding and applying the principles of engineering thinking, everyone can enhance their ability to solve problems, innovate, and contribute to building a better future.
Engineering thinking involves systematic problem-solving and critical thinking skills that are invaluable in everyday life. This article explores why these skills are essential for everyone.
Understanding Engineering Thinking
What is Engineering Thinking?: Engineering thinking is a systematic approach to problem-solving that draws on principles of engineering to address complex issues efficiently and effectively. It involves critical and analytical thinking, creativity, and a structured methodology to design, test, and implement solutions.
Critical and Analytical Skills: Engineering thinking is a structured approach to problem-solving that not only addresses complex technical challenges but also develops critical and analytical skills. These skills are invaluable and can be applied to a wide range of situations beyond engineering. This article explores how engineering thinking fosters these skills and their broad applicability.
How Engineering Thinking Helps Individuals Grow and Become Smarter in Life
Engineering thinking is a powerful approach to problem-solving that equips individuals with skills and habits that foster personal growth and intelligence. This article explores how adopting engineering thinking can help individuals become smarter and more capable in various aspects of their lives.
Enhancing Problem-Solving Skills By Systematic Approach to Challenges
Structured Problem-Solving: Engineering thinking involves a structured approach to identifying and solving problems, ensuring that all aspects of an issue are considered and addressed methodically.
Example: When faced with a complex project at work, breaking it down into smaller tasks and systematically tackling each one leads to effective and efficient solutions.
Critical Thinking and Analysis
Evaluating Information: Engineering thinking requires the evaluation of data and information critically, leading to well-informed decisions.
Example: Analyzing financial statements and market trends before making investment decisions helps in choosing the best options.
Encouraging Creative Solutions
Innovative Mindset: Engineering encourages thinking outside the box to develop innovative solutions within given constraints.
Example: Designing a unique marketing strategy for a new product by combining traditional methods with innovative digital approaches.
Design Thinking
Human-Centered Design: Engineering thinking includes design thinking, which focuses on creating solutions that are both functional and user-friendly.
Example: Creating a user-friendly mobile app interface by considering user feedback and design principles.
Learning from Failure
Resilience: Engineering thinking teaches individuals to view failures as learning opportunities, fostering resilience and adaptability.
Example: Learning from a failed business venture and using the insights to start a more successful one.
Embracing Iteration and ImprovementBy Continuous Improvement: Engineering thinking involves iterating solutions based on feedback and performance, leading to continuous improvement.
Example: Improving personal fitness by regularly evaluating and adjusting workout routines based on progress and feedback.
Effective Communication with Clear and Precise Communication: Engineers learn to communicate complex ideas clearly and precisely, a skill that is valuable in any context.
Example: Presenting a well-organized report to stakeholders, clearly explaining the technical details and implications.
Collaborative Teamwork
Team Collaboration: Engineering projects often require collaboration, teaching individuals how to work effectively in teams.
Example: Leading a project team to develop a new product, ensuring that everyone’s expertise is utilized and coordinated.
Curiosity and Continuous Learning
Staying Updated: Engineering thinking fosters a mindset of curiosity and continuous learning, encouraging individuals to stay updated with the latest advancements and knowledge.
Example: Regularly attending workshops and courses to stay informed about the latest developments in one’s field.
Adapting to Technological Changes
Embracing Technology: Understanding engineering principles helps individuals adapt to and leverage new technologies effectively.
Example: Learning to use new software tools to improve productivity and efficiency in daily tasks.
Engineering thinking provides a robust framework for problem-solving, creativity, resilience, collaboration, and continuous learning. By adopting this mindset, individuals can enhance their personal and professional lives, becoming smarter and more capable in handling the challenges and opportunities they encounter. Embracing engineering thinking not only helps in achieving specific goals but also fosters overall intellectual and personal growth, leading to a more fulfilling and successful life.
The future of ontological engineering is promising, especially as the need for intelligent data integration, semantic interoperability, and advanced AI capabilities continues to grow. Here are several key factors that suggest a bright future for this field:
1.
Increased Demand for Interoperability- IoT Expansion: As the Internet of Things (IoT) expands, the need for seamless data exchange between diverse devices and systems will drive the adoption of ontological engineering.
- Data Integration: Organizations will increasingly require sophisticated data integration solutions to leverage data from various sources, making ontologies essential.
2. Advancements in AI and ML
- Enhanced AI: Ontologies can improve AI's ability to understand context and semantics, leading to more advanced and accurate machine learning models.
- Explainable AI: Ontologies can help in developing explainable AI systems by providing clear, structured representations of knowledge that can be used to explain AI decisions.
3. Growth of the Semantic Web
- Linked Data: The vision of the Semantic Web, where data is interconnected and easily accessible, relies heavily on ontologies. This will promote the growth and adoption of ontological engineering.
- Standardization: Ongoing efforts to standardize ontological languages and tools will make it easier to develop and use ontologies, furthering their adoption.
4. Industry Adoption
- Healthcare: Ontologies can play a crucial role in healthcare by enabling better data sharing, integration, and understanding of complex medical information.
- Finance: Financial institutions can use ontologies to improve data analytics, risk management, and regulatory compliance.
- Manufacturing: In manufacturing, ontologies can enhance supply chain management, product lifecycle management, and interoperability between systems.
5. Academic and Research Developments
- Research Innovations: Ongoing research in knowledge representation, reasoning, and semantic technologies will continue to advance the field.
- Education and Training: As more educational programs and resources become available, the expertise in ontological engineering will grow, fostering broader adoption.
6. Tool and Technology Improvements
- User-Friendly Tools: The development of more user-friendly and integrated tools for creating, managing, and using ontologies will lower the barrier to entry.
- Integration with AI/ML Frameworks: Better integration of ontological tools with popular AI and ML frameworks will encourage their use in AI projects.
7. Policy and Regulatory Support
- Regulatory Compliance: As regulations around data privacy, interoperability, and transparency increase, ontologies can provide structured ways to meet these requirements.
- Government Initiatives: Government initiatives promoting data sharing and interoperability in various sectors (e.g., healthcare, smart cities) will drive the adoption of ontological engineering.
Potential Challenges
- Complexity Management: Managing the complexity of large-scale ontologies will remain a challenge, requiring ongoing innovations in tooling and methodologies.
- Adoption Resistance: Overcoming resistance to adoption due to perceived overhead and the need for specialized expertise will be essential.
Conclusion:
Ontological engineering is poised to play a critical role in the future of AI, IoT, and data-driven technologies. By addressing current challenges and leveraging ongoing advancements in technology and research, the field can achieve widespread adoption and significantly impact various industries. As the need for intelligent, interoperable systems continues to grow, ontological engineering will become increasingly important, driving innovation and enabling new capabilities across diverse domains.
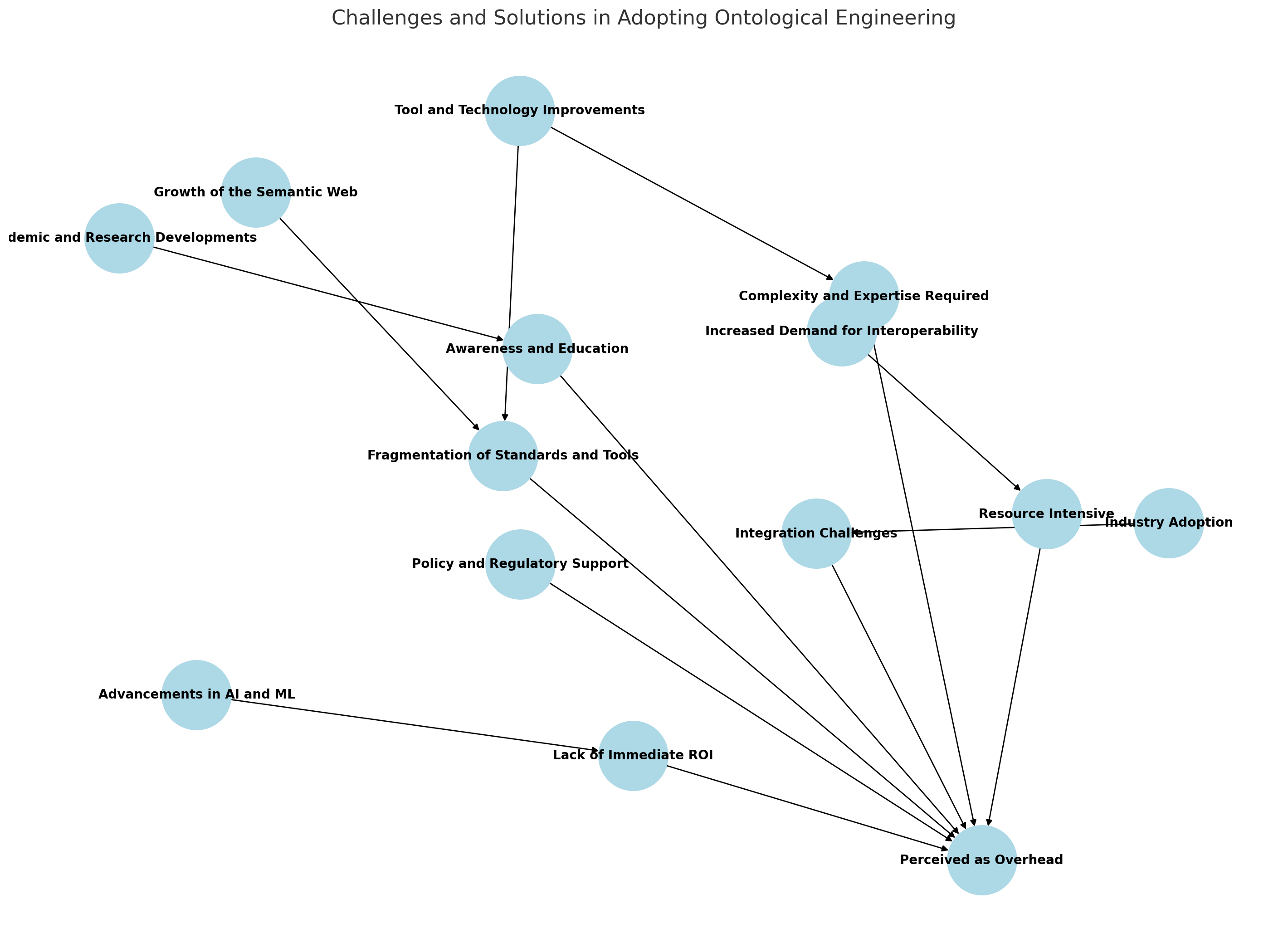
Here's a diagram that represents both the challenges and the solutions for adopting ontological engineering. The diagram illustrates how each challenge relates to the perceived overhead and how various solutions can address these challenges:
The arrows indicate how each solution can help mitigate the respective challenges, ultimately reducing the perception of ontological engineering as an overhead. This integrated view helps visualize the multifaceted approach required to overcome the barriers to adopting ontological engineering.
Ontological engineering principles are indeed powerful and beneficial for many applications, including IoT, AI, and ML-centric websites. However, there are several reasons why these principles might not be as widely adopted as one might expect:
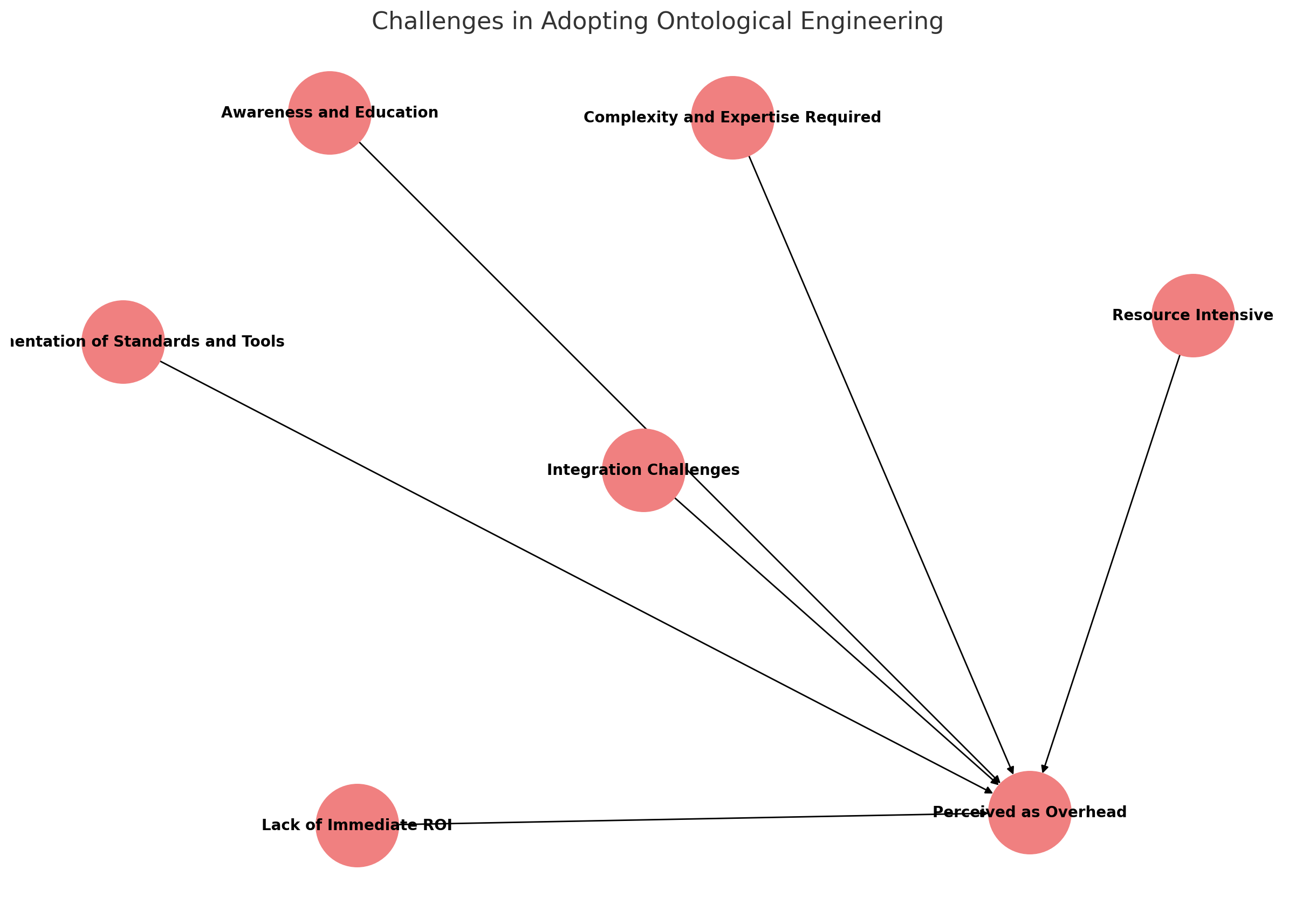
1. Complexity and Expertise Required
- Steep Learning Curve: Developing and maintaining ontologies require specialized knowledge and skills in formal logic, knowledge representation, and domain-specific expertise.
- Technical Expertise: Implementing ontologies often involves complex tools and languages (e.g., OWL, RDF), which can be a barrier for developers who are more familiar with traditional programming languages and frameworks.
2. Resource Intensive
- Time-Consuming: Creating detailed and comprehensive ontologies can be a time-consuming process, requiring significant effort in analysis, design, and validation.
- Costly: The development and maintenance of ontologies can be costly in terms of both human resources and computational resources.
3. Lack of Immediate ROI
- Long-Term Benefits: The benefits of ontological engineering, such as improved data integration and enhanced AI capabilities, often materialize in the long term. Many organizations prioritize short-term gains and quick wins, leading to less investment in ontology development.
- Unclear Immediate Impact: For some projects, the immediate impact of using ontologies may not be clear, making it hard to justify the investment to stakeholders.
4. Fragmentation of Standards and Tools
- Diverse Standards: The field of ontological engineering involves various standards and tools, which can be confusing and lead to fragmented efforts. This lack of a unified approach can discourage adoption.
- Tooling Issues: While there are tools like Protégé for ontology development, they might not be as user-friendly or well-integrated with mainstream development environments and workflows.
5. Integration Challenges
- Legacy Systems: Many organizations have legacy systems with data that are not designed for semantic interoperability. Integrating ontological approaches with these systems can be challenging and require significant re-engineering.
- Data Silos: Data silos within organizations can impede the effective implementation of ontologies, as data needs to be shared and linked across different departments and systems.
6. Awareness and Education
- Lack of Awareness: Many developers, data scientists, and decision-makers might not be fully aware of the benefits and capabilities of ontological engineering.
- Educational Gaps: There is a need for more educational resources and training programs to bridge the knowledge gap and promote the adoption of ontological principles.
7. Perceived as Overhead
- Initial Overhead: The initial effort required to develop and implement ontologies is often seen as overhead compared to more straightforward, immediate solutions.
- Perceived Complexity: The perceived complexity of ontological engineering can deter teams from adopting these practices, especially when simpler alternatives are available.
Addressing the Challenges
To promote the adoption of ontological engineering principles in IoT and AI/ML-centric websites, several steps can be taken:
- Education and Training: Increase awareness and provide training on the benefits and implementation of ontological engineering.
- Tool Development: Develop more user-friendly tools and frameworks that integrate well with existing development environments.
- Standardization: Promote standardization efforts to reduce fragmentation and provide clear guidelines and best practices.
- Showcase Success Stories: Highlight successful case studies and examples where ontological engineering has provided significant benefits.
- Collaboration: Encourage collaboration between academia, industry, and standardization bodies to drive innovation and adoption.
By addressing these challenges, the principles of ontological engineering can become more mainstream and widely adopted, leading to more intelligent, interoperable, and effective IoT and AI/ML-centric systems.
Ontology engineering is considered a futuristic engineering field that complements and extends the capabilities of artificial intelligence (AI) for several reasons:
1.
Enhanced Data Interoperability- Data Integration: Ontology engineering allows for the integration of data from diverse sources by providing a shared vocabulary and structure. This interoperability is crucial for AI systems, which often require access to vast amounts of heterogeneous data.
- Standardization: By creating standardized ontologies, different systems can communicate and understand each other’s data, facilitating seamless data exchange and collaboration.
2.
Improved Knowledge Representation- Semantic Understanding: Ontologies provide a formal way to represent knowledge in a specific domain, including the relationships between concepts. This enhances the ability of AI systems to understand and reason about data contextually.
- Complex Relationships: Ontologies can model complex relationships and rules within a domain, enabling AI systems to perform more sophisticated reasoning and inference.
3.
Facilitation of Semantic Web- Linked Data: Ontology engineering is a key component of the Semantic Web, which aims to create a web of data that can be easily shared and reused. This makes data more accessible and usable by AI applications, driving innovation and development in the field.
- Machine-Readable Data: By structuring data in a way that is understandable by machines, ontologies enable more advanced AI applications, such as intelligent agents and automated decision-making systems.
4.
Enhanced AI Capabilities- Contextual Understanding: Ontologies provide context to data, allowing AI systems to understand not just the data itself but also its meaning and relationships. This leads to more accurate and meaningful AI-driven insights and actions.
- Knowledge Sharing: Ontologies facilitate the sharing and reuse of knowledge across different AI systems, promoting more robust and comprehensive AI models.
5.
Support for Advanced Applications- Domain-Specific AI: Ontology engineering enables the development of domain-specific AI applications by providing tailored knowledge representations. This is particularly important in fields like healthcare, finance, and legal, where domain knowledge is complex and critical.
- Semantic Search and Discovery: Enhanced search capabilities powered by ontologies allow for more precise and relevant information retrieval, improving the effectiveness of AI-driven search engines and recommendation systems.
6.
Improvement in Human-Machine Interaction- Natural Language Processing (NLP): Ontologies enhance NLP by providing structured knowledge that helps AI understand and generate human language more accurately.
- Personalization: By understanding the context and relationships between concepts, AI systems can provide more personalized and context-aware recommendations and services.
7.
Future-Proofing AI Systems- Scalability: Ontologies make it easier to scale AI systems by providing a clear and structured way to add new knowledge and relationships as the domain evolves.
- Adaptability: Ontologies allow AI systems to adapt to new domains and contexts more easily, ensuring they remain relevant and effective in a rapidly changing technological landscape.
ConclusionOntology engineering is not just another futuristic engineering field; it is a foundational technology that enhances and extends the capabilities of AI. By providing structured, semantic representations of knowledge, ontologies enable AI systems to understand, reason, and interact with data in more advanced and meaningful ways. This synergy between ontology engineering and AI paves the way for more intelligent, interoperable, and context-aware systems, driving the future of technology and innovation.