Ontological engineering principles are indeed powerful and beneficial for many applications, including IoT, AI, and ML-centric websites. However, there are several reasons why these principles might not be as widely adopted as one might expect:
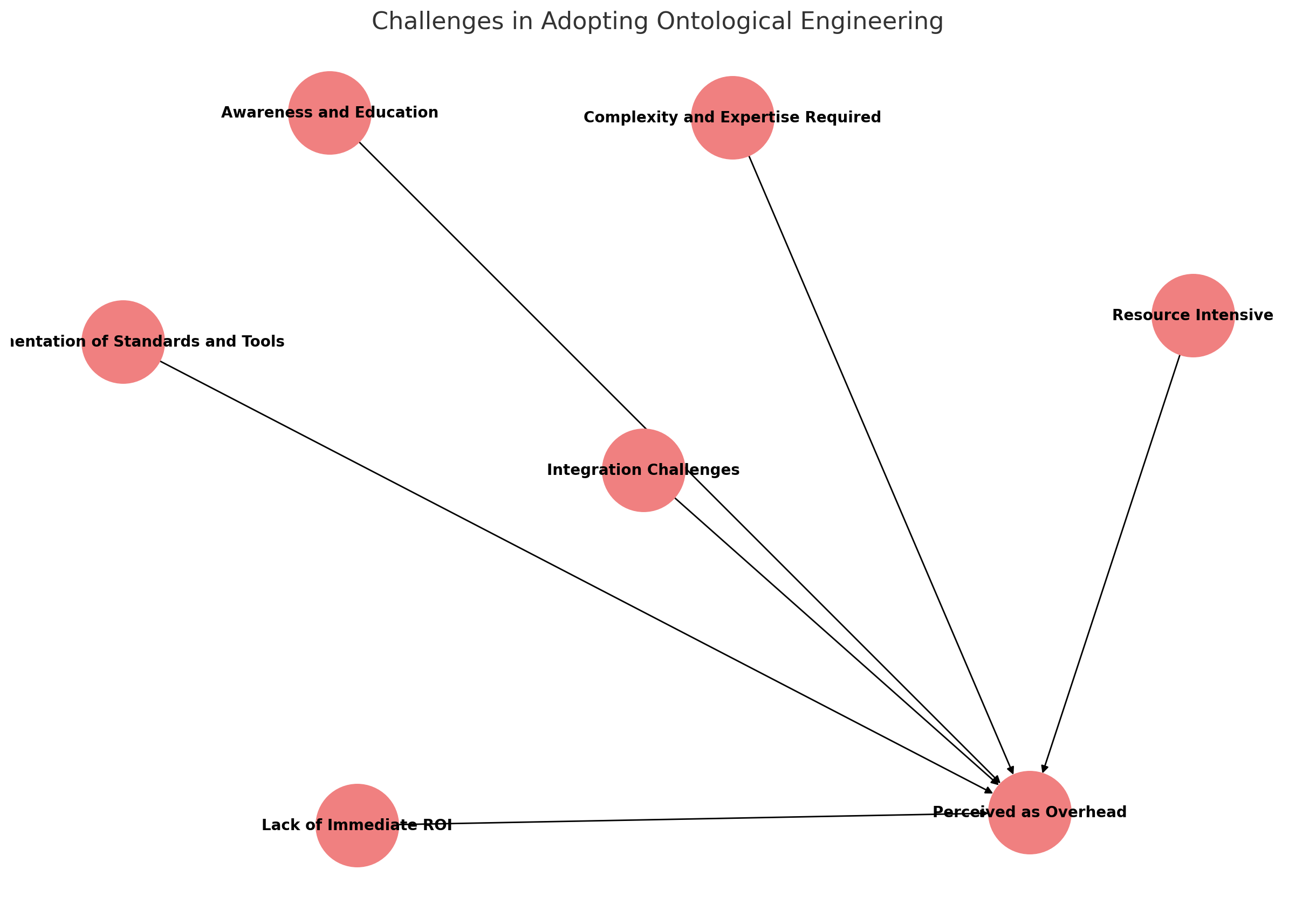
1. Complexity and Expertise Required
- Steep Learning Curve: Developing and maintaining ontologies require specialized knowledge and skills in formal logic, knowledge representation, and domain-specific expertise.
- Technical Expertise: Implementing ontologies often involves complex tools and languages (e.g., OWL, RDF), which can be a barrier for developers who are more familiar with traditional programming languages and frameworks.
2. Resource Intensive
- Time-Consuming: Creating detailed and comprehensive ontologies can be a time-consuming process, requiring significant effort in analysis, design, and validation.
- Costly: The development and maintenance of ontologies can be costly in terms of both human resources and computational resources.
3. Lack of Immediate ROI
- Long-Term Benefits: The benefits of ontological engineering, such as improved data integration and enhanced AI capabilities, often materialize in the long term. Many organizations prioritize short-term gains and quick wins, leading to less investment in ontology development.
- Unclear Immediate Impact: For some projects, the immediate impact of using ontologies may not be clear, making it hard to justify the investment to stakeholders.
4. Fragmentation of Standards and Tools
- Diverse Standards: The field of ontological engineering involves various standards and tools, which can be confusing and lead to fragmented efforts. This lack of a unified approach can discourage adoption.
- Tooling Issues: While there are tools like Protégé for ontology development, they might not be as user-friendly or well-integrated with mainstream development environments and workflows.
5. Integration Challenges
- Legacy Systems: Many organizations have legacy systems with data that are not designed for semantic interoperability. Integrating ontological approaches with these systems can be challenging and require significant re-engineering.
- Data Silos: Data silos within organizations can impede the effective implementation of ontologies, as data needs to be shared and linked across different departments and systems.
6. Awareness and Education
- Lack of Awareness: Many developers, data scientists, and decision-makers might not be fully aware of the benefits and capabilities of ontological engineering.
- Educational Gaps: There is a need for more educational resources and training programs to bridge the knowledge gap and promote the adoption of ontological principles.
7. Perceived as Overhead
- Initial Overhead: The initial effort required to develop and implement ontologies is often seen as overhead compared to more straightforward, immediate solutions.
- Perceived Complexity: The perceived complexity of ontological engineering can deter teams from adopting these practices, especially when simpler alternatives are available.
Addressing the Challenges
To promote the adoption of ontological engineering principles in IoT and AI/ML-centric websites, several steps can be taken:
- Education and Training: Increase awareness and provide training on the benefits and implementation of ontological engineering.
- Tool Development: Develop more user-friendly tools and frameworks that integrate well with existing development environments.
- Standardization: Promote standardization efforts to reduce fragmentation and provide clear guidelines and best practices.
- Showcase Success Stories: Highlight successful case studies and examples where ontological engineering has provided significant benefits.
- Collaboration: Encourage collaboration between academia, industry, and standardization bodies to drive innovation and adoption.
By addressing these challenges, the principles of ontological engineering can become more mainstream and widely adopted, leading to more intelligent, interoperable, and effective IoT and AI/ML-centric systems.