The future of ontological engineering is promising, especially as the need for intelligent data integration, semantic interoperability, and advanced AI capabilities continues to grow. Here are several key factors that suggest a bright future for this field:
1.
Increased Demand for Interoperability- IoT Expansion: As the Internet of Things (IoT) expands, the need for seamless data exchange between diverse devices and systems will drive the adoption of ontological engineering.
- Data Integration: Organizations will increasingly require sophisticated data integration solutions to leverage data from various sources, making ontologies essential.
2. Advancements in AI and ML
- Enhanced AI: Ontologies can improve AI's ability to understand context and semantics, leading to more advanced and accurate machine learning models.
- Explainable AI: Ontologies can help in developing explainable AI systems by providing clear, structured representations of knowledge that can be used to explain AI decisions.
3. Growth of the Semantic Web
- Linked Data: The vision of the Semantic Web, where data is interconnected and easily accessible, relies heavily on ontologies. This will promote the growth and adoption of ontological engineering.
- Standardization: Ongoing efforts to standardize ontological languages and tools will make it easier to develop and use ontologies, furthering their adoption.
4. Industry Adoption
- Healthcare: Ontologies can play a crucial role in healthcare by enabling better data sharing, integration, and understanding of complex medical information.
- Finance: Financial institutions can use ontologies to improve data analytics, risk management, and regulatory compliance.
- Manufacturing: In manufacturing, ontologies can enhance supply chain management, product lifecycle management, and interoperability between systems.
5. Academic and Research Developments
- Research Innovations: Ongoing research in knowledge representation, reasoning, and semantic technologies will continue to advance the field.
- Education and Training: As more educational programs and resources become available, the expertise in ontological engineering will grow, fostering broader adoption.
6. Tool and Technology Improvements
- User-Friendly Tools: The development of more user-friendly and integrated tools for creating, managing, and using ontologies will lower the barrier to entry.
- Integration with AI/ML Frameworks: Better integration of ontological tools with popular AI and ML frameworks will encourage their use in AI projects.
7. Policy and Regulatory Support
- Regulatory Compliance: As regulations around data privacy, interoperability, and transparency increase, ontologies can provide structured ways to meet these requirements.
- Government Initiatives: Government initiatives promoting data sharing and interoperability in various sectors (e.g., healthcare, smart cities) will drive the adoption of ontological engineering.
Potential Challenges
- Complexity Management: Managing the complexity of large-scale ontologies will remain a challenge, requiring ongoing innovations in tooling and methodologies.
- Adoption Resistance: Overcoming resistance to adoption due to perceived overhead and the need for specialized expertise will be essential.
Conclusion:
Ontological engineering is poised to play a critical role in the future of AI, IoT, and data-driven technologies. By addressing current challenges and leveraging ongoing advancements in technology and research, the field can achieve widespread adoption and significantly impact various industries. As the need for intelligent, interoperable systems continues to grow, ontological engineering will become increasingly important, driving innovation and enabling new capabilities across diverse domains.
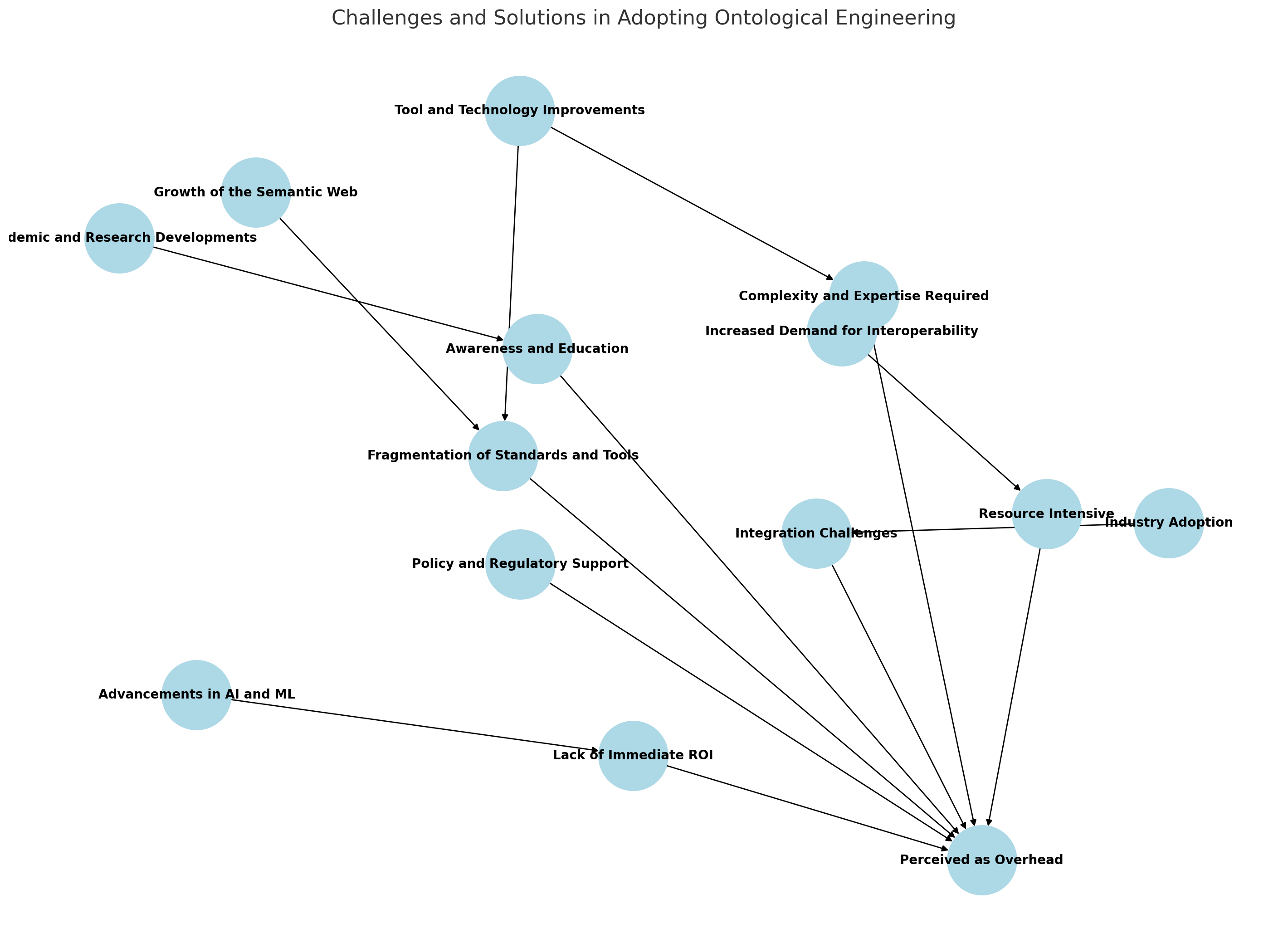
Here's a diagram that represents both the challenges and the solutions for adopting ontological engineering. The diagram illustrates how each challenge relates to the perceived overhead and how various solutions can address these challenges:
The arrows indicate how each solution can help mitigate the respective challenges, ultimately reducing the perception of ontological engineering as an overhead. This integrated view helps visualize the multifaceted approach required to overcome the barriers to adopting ontological engineering.